Bayes theorem delivers representative samples at a significantly lower cost. So if its better and more informative, and commercially useful, why not do it this way?
Before you are three doors. Behind one is a shiny sports car and behind the other two are goats. Whichever you choose, you have a 33% random chance of winning the car… After choosing, one of the other doors is opened to reveal a goat. Do you stick with your door or switch? Does it matter? Well, that depends on how much you like goats.
This is the Monty Hall problem. To improve the odds of winning, you must switch. This is because you learnt where one of the goats was hiding. The problem changed. You received more data. The same is true of fielding surveys.
Having all but consigned random methodologies to history, we take care in getting as close to randomness as budgets allow. We accept that our estimates cannot be exact so calculate a theoretical range within which we expect each estimate to fall 95 times, if the survey were repeated 100 times. Similarly, the greater the difference between two estimates the more confident we are that the difference exists in reality. When large enough, we claim statistical significance.
In fairness, this assumes that we have a pure random sample, which we don’t. In the same breath, the customary 95% confidence interval isn’t a high bar either. By definition, we will still see the same result 1 in 20 times, even when no real difference exists. To guarantee interesting results, an unscrupulous researcher need only gently clean inconvenient data, or stop surveying when significant differences emerge by chance. It’s easy to cook insights out of noise, to obliterate companies with a string of bogus recommendations underpinned by seemingly pleasing evidence.
This Frequentist approach is clunky and unintuitive; the above trickery only one of the many ways it can be browbeaten. However, it is not wrong. It is the empirical bedrock beneath social science, without which survey research is nothing more than a shake of a Magic 8 Ball.
Nonetheless, we are too accustomed to our samples approximating randomness and the market having a true, fixed, yet unknowable answer. There is a better perspective however – turn everything upside down. In Bayesian statistics, our answer is a percentage likelihood. The market is probabilistic and the sample we’ve collected is fixed. After all, there is nothing random about data once collected, just as there is no randomness to a coin toss after the coin lands.
While Frequentists compel you to withhold judgement until all the data is in, Bayesians appreciate that you may already have reasoned opinions that may bend as more information is introduced. In this way, Bayesians mimic learning. Data that differs from expectation reduces confidence – consistency naturally increases it. Bayes’s theorem plays an important role in AI and A/B testing, but how might surveys operate?
Fieldwork might be painfully slow, especially during early stages. This is one of the few downsides, outweighed by many upsides.
We would discard awkward hypothesis testing in favour of the language of decision-making, able to be ‘95% confident’, rather than being ‘95% confident our result lies within a specified margin of error’.
We would rarely work from scratch, hard-wiring previous findings into fresh research. This added stability allows for smaller samples. Likewise, we would never require more interviews than necessary to achieve the confidence we sought, placing quality over quantity while slashing the cost of any research that allows for structured exploration, such as brand trackers, satisfaction monitors and campaign tests.
Taken to the utmost extreme, we would no longer seek representative quotas for their own sake, when real time data shows we are confident without. This offends Frequentist sensibilities while being entirely plausible and demonstrable, a twist on another famed puzzle efficiently solved by Bayesians, the multi-armed bandit.
I proudly add that Reverend Bayes rests in the same cemetery as my great-great-great-grandfather but in doing so must concede that Bayes’s theorem is hardly new. Survey research is Frequentist for historical and practical reasons and until recently the above conjecture was unworkable – laughably so.
UCL/YouGov’s polling model is a blistering example to the contrary, set upon the most complex and grandest of stages. It encourages the rest of us to revisit our tenets. As we feel the economics of survey research shift beneath our feet, the moment to do so looms. Ultimately, the urgency with which we switch perspective will depend on how much we like goats.
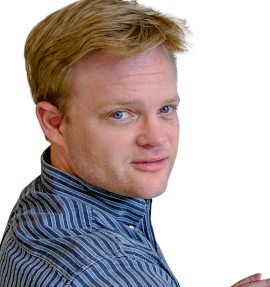
I am a marketing scientist with 24 years of experience working with sales, media spend, customer, web & survey data. I help brands and insight agencies around the world get the most out of data, by combining traditional statistics with the latest innovations in data science. Follow me on Linkedin for more of this sort of thing.