Before Explainable ML, a market researcher would be laughed off the stage if they presented a machine learning model as insight. How long until they are laughed off because they don't have ML?
Were my six-year-old self to stumble across a new swear word, my mother would threaten to put mustard on my tongue. This sent me screaming around the house , my little arms flailing in terror. Silly as it seems now, I have levelled the same at my own students, should they suggest that correlation implies causation. To a statistician, there are few ideas more profane.
Interpreting data in this way means that you live in a world where ice-cream causes drowning and washing your car causes it to rain. In reality, we buy ice-cream and swim at the same time of year and, sometimes, it rains. No matter how intuitive or consistent correlations appear, they are invitations to overestimate, to claim insight where there is none or misinterpret reality entirely.
As humans, we naturally want to think in these terms, and as researchers, we want to explain why things happen. So, what does it mean to cause something? Philosophy lends us a useful trick – the study of counterfactuals. The counterfactual framework of causal inference assumes that an individual can have many causal states with more than one potential outcome at a time. The difference between what might have happened and what actually happened is inferred to be the cause. Simple enough, right? No. Not really.
For example, can we prove our sponsorship campaign causes sales to increase? To isolate causality, we need enough information to answer some ‘What happens if…?’ questions about both the customer and campaign.
At an individual level, we might observe a customer buying our product after being exposed to the campaign. Our first counterfactual is that the customer would have bought our product regardless. Counterfactuals are the things that could have happened but didn’t.
Both actual and counterfactual outcomes are conditional on the specifics of the customer, pricing, competitor activity – a moment in time – too many conditions for a matched test and control experiment. Though, it stands to reason that if we have enough observations of both outcomes under enough conditions in enough combinations, we may yet shape a convincing case at an aggregate level. This is different from citing mere association. Rather, all other things being equal, the probability of purchasing does not increase but for the campaign.
Starting out with this everyday common sense, counterfactual thinking becomes complex disconcertingly quickly.
Causal inference relies heavily on identifying and adjusting of effects, in a world where it is impossible to account for everything and nothing remains constant. Just as with statistical inference, we are dealing in hypotheticals. Though lacking empirically, it is a pragmatic framework capable of addressing causality.
Quasi-experimental counterfactual thinking in its various guises, most notably propensity score matching, has been part and parcel of marketing science for decades. However, as machine learning ingratiates itself, it has received renewed interest and I’ve spent most of the past two years enthralled with it.
Unlike traditional modelling, machine learning’s unrestrained mathematical violence produces black box models. Black boxes are anathemas to insight professionals who want evidence to believe and a hands-on way to engage and share, before even feigning interest in prediction. Our insights are the relationships algorithms discover, not what they spit out. Confronted with black boxes, our role is diminished in modern marketing.
Counterfactuals are our crowbar into these boxes, for what is any machine-made algorithm but layer upon layer of conditions, packaged as a ‘what happens if’ calculation.
Forensic data science has codified counterfactuals, a gift to those that need to be transparent and human friendly. Picture if you will, Jack Bauer-like algorithms, interrogating models by pursing combinations, challenging relationships, manipulating, imputing and contrasting, shaking the box into confession. This is counterintelligence by machines into machines.
These confessions allow us to quantify causal drivers indirectly and map how different measures interact with each other. We can audit and debug, then demonstrate that our models make sense and get buy-in for them. Where once we were hostage to impenetrable prediction engines, explainable machine learning will now emphasise our strengths as translators and storytellers.
By the age of 10, I knew all the useful expletives. My dear Mum had no option but to make good on her promise. It backfired spectacularly – hopefully my relief and surprise reflects how you feel about data science today.
“Mmmm…” I thought, as I gestured for more, “this is pretty good stuff. I fancy using it everywhere.”
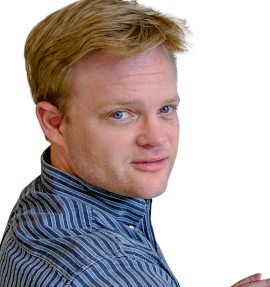
I am a marketing scientist with 24 years of experience working with sales, media spend, customer, web & survey data. I help brands and insight agencies around the world get the most out of data, by combining traditional statistics with the latest innovations in data science. Follow me on Linkedin for more of this sort of thing.